Data Warehouse Testing Tool
10 min read
A typical data warehouse (DWH) processes and stores millions of records. Hence it requires a unique data centric approach towards testing. In this article we will discuss data warehouse testing concepts, testing tools, testing challenges, test automation and testing strategies.
What is Data Warehouse Testing?
Data warehouse testing is a process of verifying data loaded in a data warehouse to ensure the data meets the business requirements. This is done by certifying data transformations, integrations, execution, and scheduling order of various data processes.
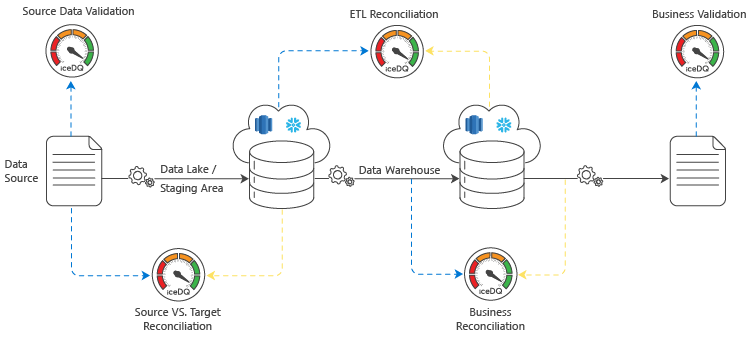
The data warehouse is loaded by data pipelines that are orchestrated in a logical sequence. Hence much of data warehouse quality depends on the data transformation processes. Please refer ETL testing as it is essential to the understanding of data warehouse testing.
What is a Data Warehouse Test Automation Tool?
Conventional Testing tools are designed for UI based applications. A data warehouse testing tool is purpose-built for data centric systems and designed to automate data warehouse testing and certification. It is used during the development phase of DWH.
For example, iceDQ is used for data warehouse testing. It can test both data processes and data in a DW. Let’s jump into the concepts. |
What is a Data Warehouse Test Automation Tool?
Conventional Testing tools are designed for UI based applications. A data warehouse testing tool is purpose-built for data centric systems and designed to automate data warehouse testing and certification. It is used during the development phase of DWH.
For example, iceDQ is used for data warehouse testing. It can test both data processes and data in a DW. Let’s jump into the concepts. |
Data Warehouse Testing Concepts
A typical data warehouse doesn’t have a graphical user interface (GUI); instead, it has thousands of batch jobs processing data every night. Data reconciliation and data validation are the two ways to test a data warehouse.
Data Validation: In this method, business rules are applied to validate and test the data. |
|
Data Reconciliation: In this method, testing is done by comparing the data in warehouse with the source data. |
Data Validation: In this method, business rules are applied to validate and test the data. |
Data Reconciliation: In this method, testing is done by comparing the data in warehouse with the source data. |
Data Warehouse Testing Types
Data warehouse testing is accomplished at different levels of success with manual, script based, and automated testing.
- Manual Data Warehouse Testing: This involves visual inspection of the data and is totally manual.
- Script based Data Warehouse Testing: Here testers create Ad-hoc scripts in java or python to test the data warehouse.
- Automated Data Warehouse Testing: It is the only approach that provides 100% test automation and enables test at scale with very good test coverage.
Challenges in Data Warehouse Testing
Data warehouse testing has many challenges to overcome because it is fundamentally different from application testing and is also relatively new. Here are some of the key challenges:
- Large Data Volume: Manual data testing is impossible because humans cannot deal with more than a few hundred records. Some data warehouse testing tools test their data by first storing data in a temporary database, such tools cannot scale and do not work for large volumes of data.
- Different Data Sources: By its very nature a data warehouse has multiple data sources, different database vendors, and different data formats. Since the source database is different from the data warehouse it is very difficult to compare data across two systems. For each source the datatypes are different and so are the flavors of their SQL dialects.
- Multiple file Formats as Source: If the data is received in files, then it adds another layer of complexities. Since it is not possible to analyze the data in a file with SQL, advanced scripting is required.
- Number of Data Processes: In a typical data warehouse project there are thousands of data processes. It is impossible to create manual test scripts for each of these data processes.
- Process Orchestration: There is scheduling and orchestration of various interdependent data jobs that define the final data in a data warehouse. This requires equivalent testing effort to deal with the process sequencing and scheduling complexity.
- Data Integration: A data warehouse typically integrates multiple records or updates one record from multiple data sources. It becomes very complex to test such conditions.
- Data Warehouse Regression Testing: Even if the data is in production, changes in business often result in changes of the data processing patterns. These changes require regression testing of the data warehouse.
- Dynamic Nature of Data: Unlike application screens, data warehouse testing is not static. The testing totally depends on the input data. Every time the data warehouse is loaded the input data changes and hence, the output. This makes testing very difficult.
- Incremental Load: Testing incremental load is difficult as the dataset needs dynamic conditions to choose only a specific part of the data at runtime of each test. Usually this is based on transactions and entry dates.
- Exception Reports: Just passing and failing a data test does not help. The test must be able to provide the exact row and column location of the data issue as it is needed for defect resolution.
Automated Data Warehouse Testing
The challenges mentioned above make it necessary to use a purpose-built automated tool such as iceDQ for data warehouse testing.
Let’s have a quick look at the capabilities necessary for data warehouse test automation tool.
- Scalable Processing: The data volumes in a data warehouse can go beyond terabytes. Since you are essentially testing data, you need software with a scalable in-memory engine and cluster processing with no data limits.
- Database Connectors: For data testing between source and the data warehouse the tool should support comparison across multiple databases. For example, iceDQ has support for 100+ database vendors and their associated SQL versions.
- File Connectors: The tool should support text, JSON and many other data file formats.
- Test Across Data Source: For testing data is compared between two databases. The achieve this, must have the capabilities to connect across two databases at the same time.
- Low-code/No-code: It needs a low-code, no-code approach to accelerate data warehouse testing.
- Complex Data Transformations: Support complex rules to verify data transformation and data integration.
- Centralized Rules Repository: A central repository to store all the tests created by the QA team. This removes dependencies on developers and testers due to attrition.
- Asynchronous Test Executions: Often testers get locked out of their desktops due to long running tests, thus wasting precious time. Tools such as iceDQ support asynchronous execution of processes freeing up the developer.
- Test Case Organization: Built-in support for complex test organization, dependency, and scheduling.
- Regression Testing: Regression testing allows combining old with new tests to recertify the changes in the data warehouse. A data warehouse may have thousands of interdependent processes, and any small change in these processes can cause data issues in downstream systems. Therefore, a tool supporting Regression testing in a Data Warehouse will ensure that new code changes will not break old functionality, and at the same time, the new feature is working as expected.
- Dynamic Parameters: iceDQ supports dynamic parameters in the test that takes care of incremental data loads and other issues.
- Exception Reports: The data warehouse testing must not only provide magnitude of failure but also the exact row and column of the failure. For example, iceDQ has data exception reports for this purpose.
- Management Reporting: The tool must support management and compliance reporting.
- Integrations: The ability to integrate is vital in an enterprise landscape. Organizations have many tools such as test management, defect management, scheduling, and notifications. They want to integrate data warehouse test automation tools such as iceDQ with others to achieve end-to-end automation. The tool must be able to report and log defects in Test case management tools such as JIRA, TLM, etc.
Data Warehouse Testing Strategy
For successful DWH testing, the strategy must include the following plan involving both technical as well as business users.
- Identify SMEs: Identify the SMEs who can explain the business process and data as well.
- Data Sources: Identify all the data sources feeding into the data warehouse. This must include new as well as current data sources.
- Source Data Model: Understand the data model of the source systems.
- Data Warehouse Model: Understanding the data warehouse data model.
- Downstream Apps: Find the downstream extracts, reports, and users.
- Process Dependency Diagram: To determine the sequence of process execution document the PDD (Process Dependency Diagram).
- Scheduling & Orchestration: Document the schedule and check for conflicts in the schedules for process orchestration.
- Data Flow Diagram (DFD): Understand the flow of data through various systems and tables with the help of DFD.
- Reference Data: Document the reference data and the list of values. Ensure the actual reference data is mapped to standard reference values.
- Data Mapping Document: Use the data mapping document to study the data mapping and data transformation rules. These are useful for the creation of data testing rules.
- Test Cases: Based on the business requirements and the mapping document, create test cases.
- Test Rules: Create actual executable testing rules and link them to test cases.
- Schedule and execute Rules: Run the test rules to get results.
- Analyze Results: Understand the results so that developers can fix the processes.
- Report: Generate execution reports as well as summary reports.
Now that we understand the data warehouse testing strategy lets dig into the testing technicalities of a data warehouse.
How to Test Data Warehouse?
Testing a data warehouse is a combination of steps executed systematically. It is not a single task, but a variety of tasks orchestrated while applying different testing methods. The steps are mentioned below.
The exact techniques are mentioned below for each of the above steps.
- Test Reference Data: In a data warehouse it is very important to identify reference values. Example, Customer types (individual, corporate, government). The sudden appearance of a new reference value can put the processing logic at risk because they usually rely on preidentified values for their processing logic. Hence, compare the actual list of reference values in the data warehouse to the known values from a static list.
- Test Source Data: The source data is raw material used for processing and populating the data warehouse. The source validation tests ensure that the data meets the requirements before the processing starts.
- Test Source to Stage Data: The next step is to reconcile the source data with the data loaded in the data warehouse stage area. This ensures that all the data provided by the source system was captured by the data warehouse and there was no leakage of data.
- Test Incremental Load to Stage: It is also important to ensure that incremental or delta loads are processed correctly without corrupting or overwriting the existing data.
- Test Data Transformation: The data process transforms the data based on the requirements. The data goes through multiple processes such as summarization, filtration, integration then finally updated. It is necessary to test this transformation based on data audit rules.
- Test Business Logic:
- Test Business Logic (Recon): Even if a data process is tested successfully, it doesn’t ensure that the data is loaded properly. For example, the orders are loaded correctly and so are the shipment data. But still there are possibilities that there are some shipments for which order data doesn’t exist. This is because it is possible that the data warehouse never received some of the orders’ data.
- Test Business Logic (Validation): The business validation is usually obtained from business users.
- Test Extracts: Finally, the data sent out of the data warehouse must be tested to ensure that it is populated correctly.
Data Warehouse Data Validation Techniques
Data warehouse validation requires a multi-dimensional approach towards testing. Here are a few of the techniques listed below.
Data Warehouse Unit Testing
During the development phase of a project, unit testing is performed on a data warehouse before the code is passed on to the quality assurance team. As such there is not much difference between unit testing done by developers vs. the testing done by QA teams.
When developers do unit testing, they are focused on:
|
However, there is no set limit to the scope of unit testing if developers wish to expand it. iceDQ allows developers to create data testing rules so that they don’t need to rely on QA teams.
When developers do unit testing, they are focused on:
However, there is no set limit to the scope of unit testing if developers wish to expand it. iceDQ allows developers to create data testing rules so that they don’t need to rely on QA teams. |
Data Warehouse Performance Testing
Data warehouse performance testing measures the speed at which data is loaded into a data warehouse. It is usually calculated as the time taken to process a certain number of records. This is usually a good indicator of whether something is wrong with the performance tuning of the data process.
- Inbound Performance Testing: The speed (Row/Secs) at which data is loaded in real-time and/or in batch mode.
- Outbound Performance Testing: The speed (Row/Secs) at which data is usually delivered to reports, or extracts.
Usually, a data process logs their start time, end time and Rows Processed in log table. Then iceDQ is used to create rules against the log table to get performance numbers.
Process | Process Instance ID | #Rows Processed | Start Time | End Time |
---|---|---|---|---|
Load Orders | #123 | 189,990 | 2/2/2023 01:20:00 | 2/2/2023 1:25:12 |
Load Shipment | #234 | 190,000 | 2/2/2023 2:10:00 | 2/2/2023 2:16:00 |
Here are some factors in the test that are used to understand the data warehouse performance:
Rows/Sec processed = # of Records loaded or extracted / (end DTTM -start DTTM) Secs
Compare previous average run time vs. last run time.
Identify top 10% of the processes taking max of time for execution.
Once the performance numbers are in then identify the factors for slow performance:
- Source is slow
- Data transformation is slow
- Loading is slow
Depending on the reasons, you can either tune the data transformation process or discuss with SME to implement some kind if delta strategy for incremental processing.
Data Warehouse Regression Testing
Once the data warehouse is deployed it is not all done. Whenever changes are made upstream of the data warehouse or the data warehouse, regression testing is required to test the code refactoring.
Below are some of the factors that cause refactoring of the production code:
- Defects in code.
- Changes in business logic.
- Data sources change.
The above causes alterations in the data warehouse:
- Data model or tables
- Data transformation changes in existing process
- Addition of new data processes
- Process execution sequence
- Execution schedule
- Reference data
For regression testing is done in following steps:
- Identify impacted data elements and data processes.
- Identify previous tests that were related to the impacted area.
- From that set identify the tests that are still relevant and discard the remaining.
- Create additional new tests introduced due to the changes.
- Create a regression pack by combining the old relevant and new tests.
- Execute the regression pack as required.
The above steps will ensure that the system is recertified after the changes are made in the production DW.
Data Warehouse Integration Testing
A data warehouse typically integrates data from multiple data sources to gain a unified perspective of the data. As seen below a single table is integrated with data from multiple sources.
- A single table is populated from multiple source systems or multiple tables.
- Different columns in a table get populated and from different feeds. Sometimes even a single column can get sequentially updated by different data feeds.
Data integration creates complex challenges with testing. For integration testing, multiple rules must be created and grouped together. Then the execution is orchestrated in the correct order to ensure the data is integrated correctly.
For example, the end-of-day inventory of MARKET_VALUE table gets data from multiple sources and in different order.
MARKET_VALUE | |||||
---|---|---|---|---|---|
Date | Product | EOD Inventory | EOD Price | Total Market Value | Last Update Date |
2/2/2023 | Apple TV | 10 | $160 | $16,000 | 2/1/2023 |
2/2/2023 | Apple TV | 100 | $150 | $15,000 | 2/2/2023 |
2/2/2023 | Blu-ray Player | 2 | $300 | $600 | 2/2/2023 |
For testing such a table that is integrated with data from multiple sources, a series of tests are required to test the data integration.
- Test 1: The Product and quantity from inventory table must match the product and EOD inventory in Market value table.
- Test 2: The product prices from EOD Product Price must match the EOD Market Price in the Market Value table.
- Test 3: The Total market value must match the EOD Market Price * EOD Inventory
- Test 4: Finally, the Date should match the system date.
How to test incremental load in data warehouse
The first step for testing incremental load in a data warehouse is to identify how changes in the data can be captured relative to the previous loads.
You can use the record update date or similar, to identify when the records were last updated. Once the date time is found it is just a matter of selecting records that were updated since the last tests. However, the tests need to store the last processed date. |
The first step for testing incremental load in a data warehouse is to identify how changes in the data can be captured relative to the previous loads. You can use the record update date or similar, to identify when the records were last updated. Once the date time is found it is just a matter of selecting records that were updated since the last tests. However, the tests need to store the last processed date. |
The data warehouse usually has huge amounts of data, obviously it doesn’t make sense to reprocess all the data every day. Hence, incremental loads are very common in a data warehouse. The incremental load works in the following two ways and that has a direct impact on the testing.
- Incremental load testing based on system dates.
- Incremental load testing based on data in the configuration table.
MARKET_VALUE | |||||
---|---|---|---|---|---|
Date | Product | EOD Inventory | EOD Price | Total Market Value | Last Update Date |
2/2/2023 | Apple TV | 10 | $160 | $16,000 | 2/1/2023 |
2/2/2023 | Apple TV | 100 | $150 | $15,000 | 2/2/2023 |
2/2/2023 | Blu-ray Player | 2 | $300 | $600 | 2/2/2023 |
In the above example for increment load is identified with the following SQL can be used:
Regardless of you doing data warehouse validation testing or data warehouse reconciliation, you need to limit the data used for testing.
Data Warehouse Schema Validation Tests
The Schema is a structure that holds the data. Schema changes sometimes are introduced deliberately or by accident. Changes in schema structure can cause small or very large impacts on the code.
Data Schema:
- Tables are dropped or created.
- Columns are dropped or created.
- Column data type changes.
- Column length and precision changes.
- Column constraint changes
- Primary Key
- Foreign Keys
- Unique Keys
Performance related schema:
- Replacing views with materialized views or other wise.
- Adding or removing indexes.
For schema validation test, a data architect approved schema of the data warehouse is stored in a table or a file. Then an iceDQ rule is used to reconcile the actual schema in database to the preapproved schema. Any differences are notified as defects in the schema.
Data Warehouse Pipeline Orchestration Tests:
In a batch, data processes are executed in a specific order of events. Even if the processes are correct, executing them in the wrong order will get you incorrect data. Hence, data pipeline orchestration tests must also be designed to validate the order or sequence of execution.
MARKET VALUE
PROCESS_LOG | ||||
---|---|---|---|---|
Process | Process Instance ID | #Rows Processed | Start Time | End Time |
Load Customers | #23 | 1001 | 2/2/2023 01:00:00 | 2/2/2023 1:02:32 |
Load Products | #44 | 2901 | 2/2/2023 1:10:00 | 2/2/2023 1:13:00 |
Load Orders | #123 | 189,990 | 2/2/2023 01:20:00 | 2/2/2023 1:25:12 |
Load Shipment | #264 | 190,000 | 2/2/2023 2:10:00 | 2/2/2023 2:16:00 |
As discussed earlier, a process log table usually stores the start time of each process. Depending on the processing order the dependent process cannot start before the end of the upstream process. This provides input to the iceDQ platform to validate the process sequence.
The [start datetime] of the downstream process should be greater than the [end datetime] of the upstream process. If the sequence of events is provided, it is straightforward to test pipeline orchestration.
Reference Data Testing
A data warehouse has many reference tables. For example, a PRODUCT_TYPE reference table will have reference values such as “Camera”,” TV”, and “Audio”. Usually, the reference values are a static list and not dynamically added.
Additionally, there are data transformations logic thar is dependent on the specific reference values for their processing. So, if any reference value is added, updated, or removed it can cause havoc on the data warehouse processing.
To test reference data, maintain a static list of known reference values in a list or a table. Then a reconciliation rule in iceDQ can be used to find the unknown reference values and/or missing reference values.
Data Warehouse Data Validation Tests
Data warehouse validation is used to check that the data warehouse holds valid values as per the business requirements. It is done by applying some kind of calculations to verify the values. Here are some examples of validity tests:
- Verify that the Net sales amount calculation does not break the business rule. In the example below
Net Amount = Sales Amount – Commission Amount – Tax Amount – Fee Amount – Discount Amount
ORDER | ||||||||
---|---|---|---|---|---|---|---|---|
Order No | Product | Order Quantity | Sales Amount | Tax Amount | Commission Amount | Fee Amount | Discount Amount | Net Amount |
99 | 40 Inch TV | 1 | $1000 | $60 | $0 | $5 | $935 | |
99 | Apple TV | 1 | $200 | $12 | $0 | $188 | ||
101 | Blu-ray Player | 1 | $300 | $18 | $0 | $282 | ||
102 | Camera | 1 | $3000 | $180 | $0 | $100 | $2,820 | |
102 | Battery | 5 | $100 | $6 | $0 | $50 | $96 |
In the above example when the formula is applied the Net Amount of the battery in order #102 is incorrect.
Data Warehouse Reconciliation Tests:
Data warehouse reconciliation tests are used to identify inconsistencies between two data sets within a data warehouse. Even if a data process is programmed and executed correctly, the data warehouse can still incomplete or inconsistent data into the data warehouse, if the source system does not provide complete data.
- The source system may not have all the data that is needed to load the data warehouse.
- The source system may not be able to provide the data in a timely manner.
- The source system itself might have incorrect data.
In the example, you cannot have shipment data without having orders data. Even if the order and shipment data is being loaded by two data processes. Despite having technically correct data transformation devoid of any technical defects there might be shipment for which orders don’t exist.
ORDER_DIMENSION | ||
---|---|---|
Order No | Product | Qty |
99 | 40 Inch TV | 1 |
99 | Apple TV | 1 |
101 | Blu-ray Player | 1 |
102 | Camera | 1 |
102 | Battery | 5 |
ORDER_SHIPMENT | ||||
---|---|---|---|---|
Ship No | Order No | Product | Qty | Ship Date |
S123 | 99 | 40 Inch TV | 1 | 2/2/2023 |
S123 | 99 | Apple TV | 1 | 2/2/2023 |
S333 | 101 | Blu-ray Player | 1 | 2/6/2023 |
S343 | 102 | Camera | 1 | 2/6/2023 |
S343 | 102 | Battery | 5 | 2/6/2023 |
S999 | 109 | 50mm Lens | 1 | 2/9/2023 |
Only a data reconciliation test can capture such data issues.
Data warehouse testing best practices & conclusion
Based on the arguments above we can conclude that data warehouse testing is a beast by itself, with millions of records and thousands of processes it is unlikely that someone can test manually. A data warehouse testing tool like iceDQ is essential for automated testing. Please also refer to the document that provides additional information about ETL Testing and the difference between ETL testing and Data warehouse testing.